Paper Explores Machine Learning Methods in Interpretation of (Aero)Magnetic Anomaly Maps
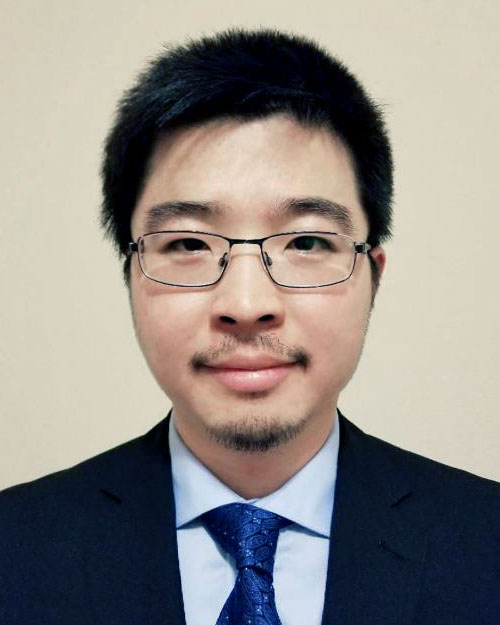
Recent work by Jiajia Sun, assistant professor in the University of Houston’s Department of Earth & Atmospheric Sciences, and his student Felicia Nurindrawati was featured on the cover of the Journal of Geophysical Research: Solid Earth. Nurindrawati is a recent EAS graduate who is now working as a machine learning engineer at TCarta.
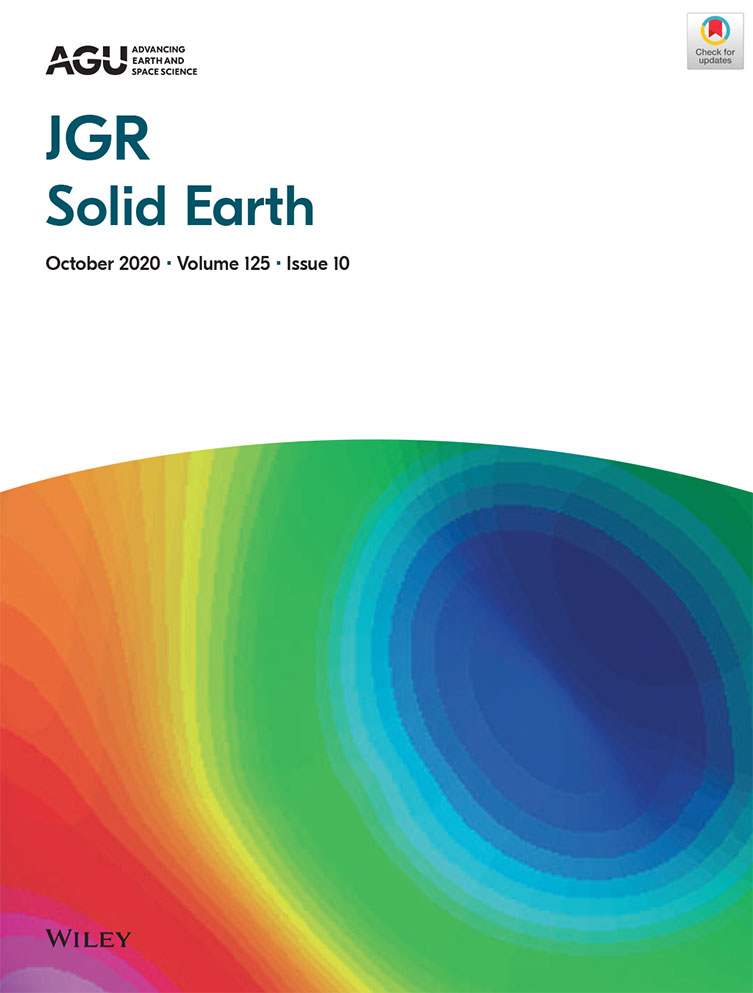
The paper was also selected as an AGU Editor’s Highlight on EOS.org. Fewer than 2% of AGU journal articles are featured in this way.
Their article, "Predicting magnetization directions using convolutional neural networks,” explores the performance of machine learning methods in the interpretation of (aero)magnetic anomaly maps. They tested various sets of convolutional neural networks (CNN) and trained the two optimal CNN designs (one for declination and one for inclination) to predict declination and inclination directly from input total field maps, without the need of reduction-to-pole, component conversions or any other wavenumber-domain computations.
“This is a big improvement as interpretation typically involves a series of steps after optimization of raw flight data. The CNN approach largely bypasses issues with classic interpretation and is anticipated to develop into a welcome addition to the existing tool kit for magnetic anomaly interpretation,” said Dr. Mark J. Dekkers, associate editor of JGR: Solid Earth, and Fellow of the American Geophysical Union.
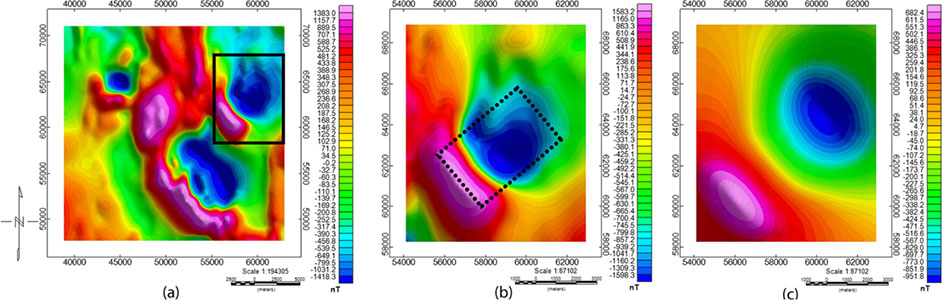
Sun explains that this is the first time that modern deep learning technique has been successfully applied to magnetic data for estimating the magnetization directions of magnetic source bodies.
“The preliminary results from our work are very encouraging, opening up doors for near real-time predictions in future,” Sun said.
The work also has profound implications for many geoscience applications, ranging from mineral exploration, to studies of oceanic plateaus, for which magnetization directions are critical pieces of information that geoscientists have been trying to extract in order to better understand the formation history of geological systems of interest.
“Considering that many of the geologically interesting and economically significant targets such as iron, copper, gold deposits, kimberlite pipes that host diamonds, etc., are magnetic, we believe that machine learning holds great potential for advancing the discovery of these targets in a more cost-effective way,” Sun said.
He noted that some of the critical minerals identified by the U.S. government, such as niobium, are also magnetic. “We see the further development of our work as not only relevant to mineral exploration but also to national security and sustainability of U.S. economy,” added Sun.
To promote reproducibility of scientific research and to stimulate future research, Sun has made their data and codes publicly available in an open data repository on Zenodo, which has already received 298 views and 333 downloads since September.