IEEE Best Land Transportation Paper (2023)
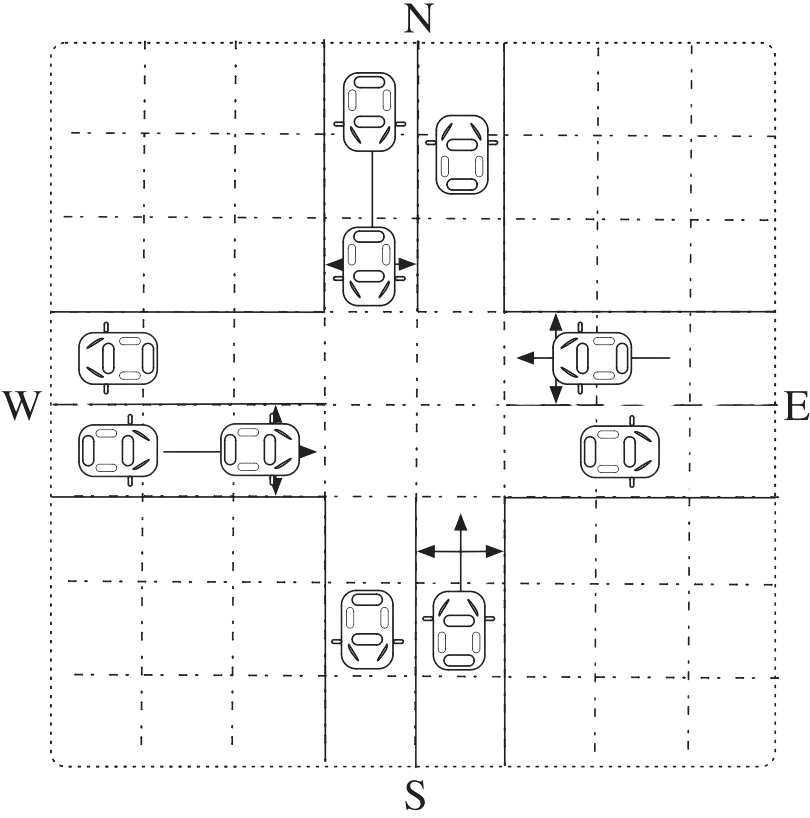
Paper: A Deep Reinforcement Learning Network for Traffic Light Cycle Control
Award: IEEE Best Land Transportation Paper Award for the year 2023
Authored by :
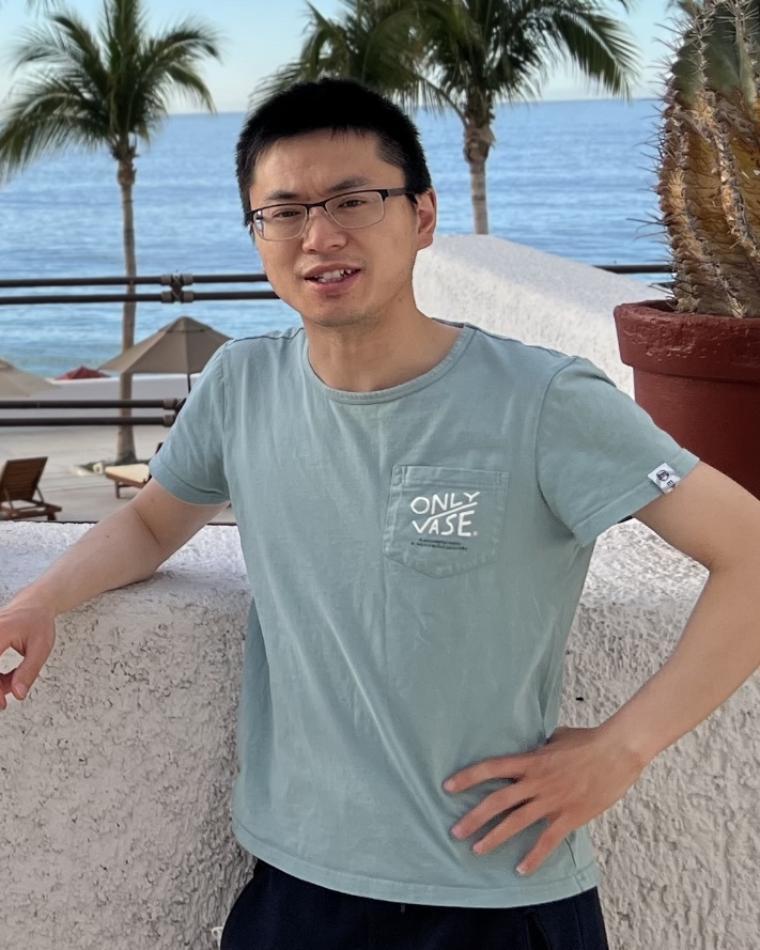
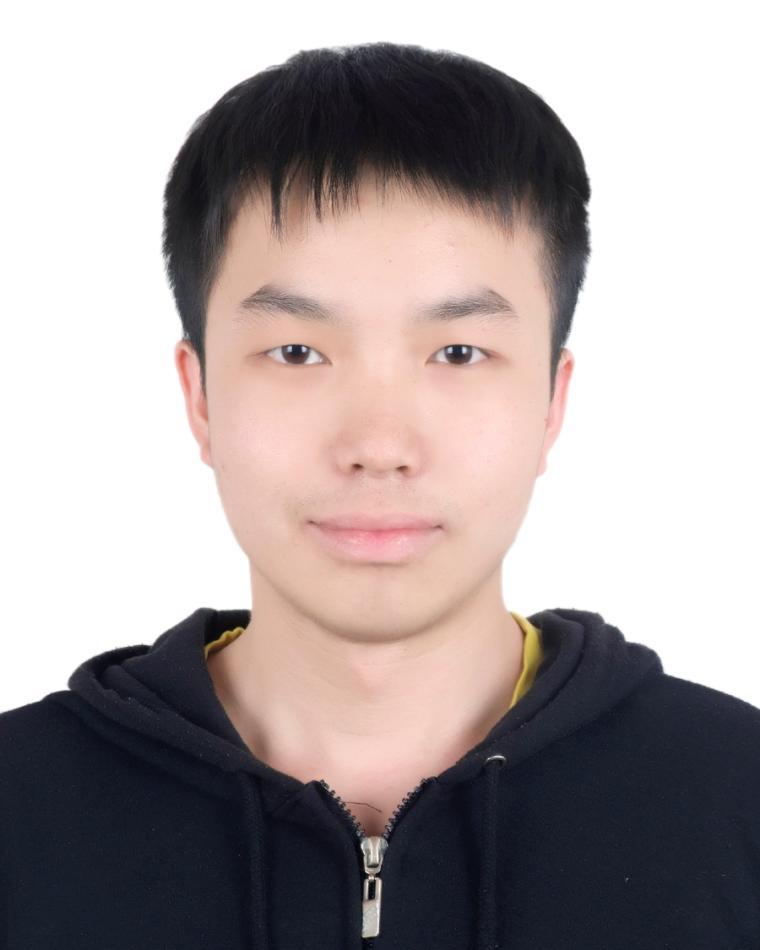
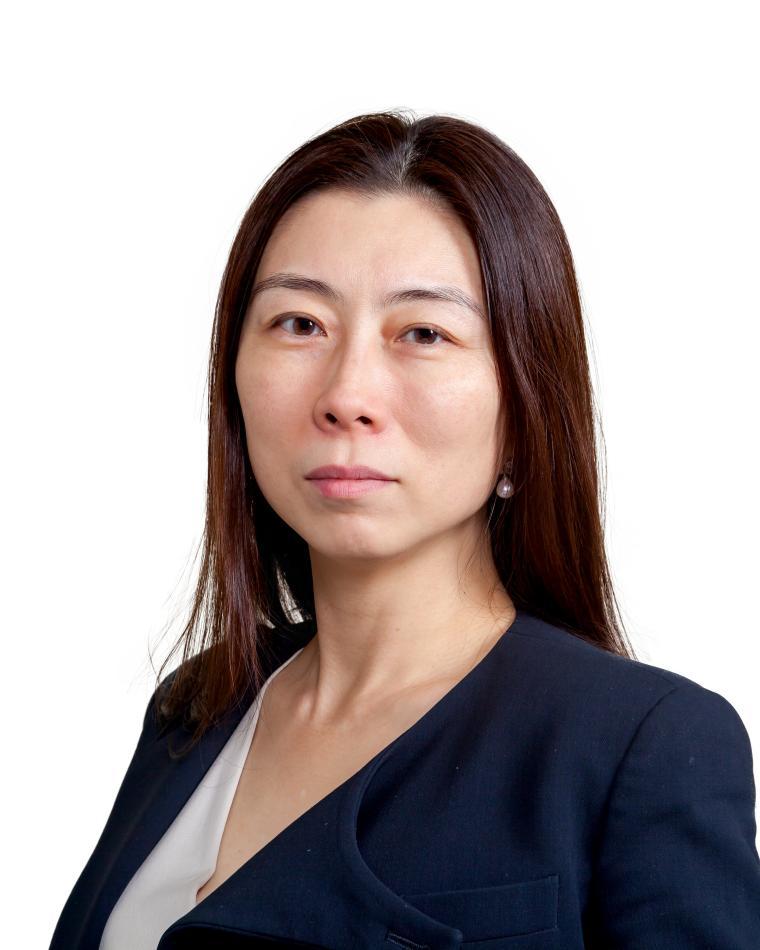
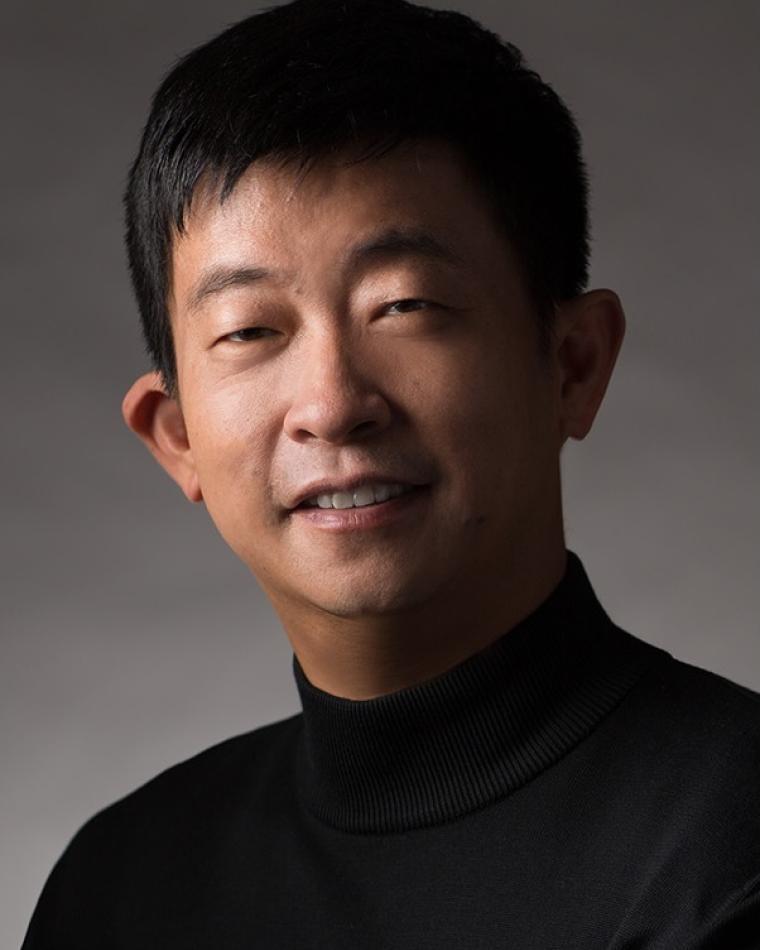
Abstract:
Existing inefficient traffic light cycle control causes numerous problems, such as
long delays and waste of energy. To improve efficiency, taking real-time traffic information
as an input and dynamically adjusting the traffic light duration accordingly is a
must. Existing works either split the traffic signal into equal duration or only leverage
limited traffic information. In this paper, we study how to decide the traffic signal
duration based on the collected data from different sensors. We propose a deep reinforcement
learning model to control the traffic light cycle. In the model, we quantify the complex
traffic scenario as stated by collecting traffic data and dividing the whole intersection
into small grids. The duration changes of a traffic light are the actions, which are
modeled as a high-dimension Markov decision process. The reward is the cumulative
waiting time difference between two cycles. To solve the model, a convolutional neural
network is employed to map states to rewards. The proposed model incorporates multiple
optimization elements to improve the performance, such as dueling network, target
network, double Q-learning network, and prioritized experience replay. We evaluate
our model via simulation on a Simulation of Urban MObility simulator. Simulation results
show the efficiency of our model in controlling traffic lights.