ICPSR Summer Workshops
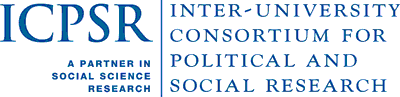
ICPSR Summer Program in Quantitative Methods of Social Research
2023 Summer Workshops by the Hobby School of Public Affairs
The Hobby School of Public Affairs announces the workshop offerings by University of Houston faculty for the 2023 Summer Program in Quantitative Methods of Social Research of the Inter-University Consortium of Political and Social Research (ICPSR).
ICPSR was launched in 1962 as a national initiative for training social scientists in research methods, including mathematical modeling, statistics, data analytics, and experimental design.
ICPSR is hosted at the University of Michigan, and runs its flagship Summer Program in Ann Arbor. The Hobby School joins a selective group of academic institutions in the ICPSR network offering summer training workshops. Other hosts of these workshops include the University of Massachusetts at Amherst, University of California at Berkeley, Indiana University at Bloomington, University of Colorado at Boulder, and the University of North Carolina at Chapel Hill. ICPSR also has three international partners: University of Glasgow, Scotland; University of St. Gallen, Switzerland; and York University, Toronto, Canada.
These workshops aim at training students and professionals in the analytical tools and software used in the analysis of data in the social sciences. The Hobby School will offer eight short workshops this summer.
- Introduction to R: May 15-19
- Introduction to Linear Regression Analysis: May 15-19
- Social Science Data and Model Visualization in R: May 22-25
- Introduction to Causal Inference: May 22-26
- Experimental Design: May 22-26
- Multilevel Analysis with R: May 22-26
- Time Series Cross-Sectional (TSCS) Data Analysis: May 30-June 2
- Intermediate Regression Analysis: May 30-June 2
For more information about the ICPSR Summer Program by the Hobby School, please contact Pablo M. Pinto (ppinto2@uh.edu), Director, Center for Public Policy, or Scott Mason (smason@uh.edu), Program Director 2 at the Hobby School.
Workshops Offered by the UH Hobby School of Public Affairs
Instructors: Ryan Kennedy, University of Houston (Department of Political Science)
Dates: May 15-19, 1:00 p.m. - 5:00 p.m. CST
Room: TBA; Hybrid
Course Description: Most students learn to use R piecemeal, by performing tasks similar to what they have already learned in another program like Stata or SPSS. The result is often frustration on the part of both students and professors. This course takes a different approach. Utilizing the "tidyverse" tools, this course shows how writing code in R can be both easy and intuitive. We will cover graphics, data management and modeling, automated updating of tables, replication using markdown, functional programming, and, time permitting, application design. This course is not about repeating in R what students have already learned elsewhere, but to instead become R programmers capable of approaching any new challenge.
Prerequisites: Basic statistics
Software: R and RStudio (both programs are free and installation instructions will be provided by instructor prior to start of workshop)
Fee: ICPSR Member - $1600; ICPSR Non-member - $2900
Introduction to Linear Regression Analysis
Instructor: Patrick Shea, University of Glasgow
Dates: May 15-19, 9:00 a.m. - 5:00 p.m. CST
Room: TBA; Hybrid
Course Description: This course is designed for participants to develop quantitative research skills with applications to social science topics. Participants will gain an overview of research design, data management, and statistical analysis and interpretations of research findings. The course will be centered around several main topics covering the basic analysis of ordinary least squares (OLS), the technique of estimating bivariate and multivariate regression models, the overall fitness of a regression equation, and the hypothesis and diagnostic testings, and more. This course takes the "learning by doing" approach by discussing the major themes in regression analysis with detailed examples, which show how the subject works in practice using Stata.
Prerequisites: All participants in this course are expected to have a working knowledge of elementary statistical concepts. We will review these concepts in the first session.
Software: n/a
Fee: ICPSR Member - $1800; ICPSR Non-member - $3300
Social Science Data and Model Visualization in R
Instructor: Boris Shor, University of Houston (Department of Political Science)
Dates: May 22-25, 8:00 a.m. - 1:00 p.m. CST
Room: TBA; Hybrid
Course Description: This course will introduce social science students to modern methods of exploring and communicating data to a variety of audiences and in a variety of formats. The primary tools will be the R programming language, RStudio as a development environment, and the tidyverse family of R packages (especially ggplot2) for visualization. The objective will be to enable students to feel confident in descriptively exploring their data, communicating that to scholarly and outside audiences, and communicating model results for presentation and paper writing purposes. Throughout, good workflow practices for practicality, efficiency, and replicability will be emphasized. Using one common set of tools, students will learn how to display visualization on the web, in papers, and presentations.
Prerequisites: n/a
Software: n/a
Fee: ICPSR Member - $1600; ICPSR Non-member - $2900
Introduction to Causal Inference
Instructor: Michael Kistner, University of Houston (Department of Political Science)
Dates: May 22-26, 1:00 p.m. - 5:00 p.m. CST
Room: TBA; Hybrid
Course Description: Since the advent of the credibility revolution, social science has paid increasing attention to issues of research design and causal identification. How can we reliably draw valid inferences? This short course introduces students to tools for thinking about and making causal inferences, with a focus on observational (i.e., quasi- or non-experimental) data. Material we will cover includes regression and matching methods, regression discontinuity designs, difference-in-differences, and synthetic control models. Emphasis will be placed on understanding why particular methods work, when each is appropriate, and best practices for how to implement them. This course is appropriate for students with a basic understanding of statistics, linear regression, and R. Classes will be a combination of lecture and working through applied examples in R, and students will leave with code they can adapt for their own research.
Prerequisites: n/a
Software: n/a
Fee: ICPSR Member - $1600; ICPSR Non-member - $2900
Experimental Design in the Social Sciences
Instructor: Blake Heller, University of Houston (Hobby School of Public Affairs)
Dates: May 22-26, 1:00 p.m. - 5:00 p.m. CST
Room: TBA; Hybrid
Course Description: This course introduces students to randomized experiments in the social sciences, aiming to help participants understand, design, and replicate randomized experiments, including lab, field, and survey experiments. We examine the process of designing experiments to test hypotheses and explore concepts of internal and external validity, level of randomization, blocking/stratification, statistical power, open science principles, and practical considerations in (and constraints to) conducting experimental research. Participants will benefit from familiarity with linear regression or having taken an introductory undergraduate statistics course, but there are no prerequisites for motivated students. Programming may be conducted in Stata or R, according to student preference. Pre-doctoral scholars or early-stage PhD students in the quantitative social sciences who are interested in integrating experiments into their future research—but have little or no prior experience designing or running experiments—would benefit from this course.
Prerequisites: n/a
Software: n/a
Fee: ICPSR Member - $1600; ICPSR Non-member - $2900
Instructor: Scott Basinger, University of Houston (Department of Political Science); Ling Zhu, University of Houston (Department of Political Science)
Dates: May 22-26, 8:30 a.m. - 12:30 p.m. CST
Room: TBA; Hybrid
Course Description: The course extends linear regression models to situations where units are nested (e.g., in schools) or clustered. We review the least squares regression framework and assumptions, then we introduce hierarchical data structures and explain how simple methods (ANOVA, clustered standard errors) were used to tackle inferential problems. The course next turns to choosing appropriate multilevel model specifications, evaluating fixed and random effects, modeling latent growth curves for longitudinal data, and selecting suitable models for panel data. After the course, students will be able to estimate and interpret random intercept and random coefficient models, analyze cross-level interactions, and visualize statistical results. Students should start with a working understanding of regression modeling. Previous experience with the R statistical computing software is helpful but lab modules will provide all the code and teach all the programming skills that students will need.
Prerequisites: n/a
Software: n/a
Fee: ICPSR Member - $1600; ICPSR Non-member - $2900
Time Series Cross-Sectional (TSCS) Data Analysis
Instructor: Ling Zhu, University of Houston (Department of Political Science)
Dates: May 30-June 2, 1:00 p.m. - 6:00 p.m. CST
Room: TBA; Hybrid
Course Description: This workshop focuses on applied econometric models for time-series cross-sectional (TSCS) data. Topics to study include specifications, estimation, and interpretation of statistical results. We will begin by reviewing the standard linear regression models and how TSCS data require additional considerations for specification and estimation. After introducing fixed effects, random effects, and mixed effects models, we will discuss additional TSCS models, including dynamic models, models with binary outcomes, and causal inference. This workshop is suitable for students from various social science fields, such as political science, economics, education, public health, and public policy. Students will learn how to explore their data to choose appropriate models and how to interpret models once estimated. Prior knowledge of regression analysis and R is helpful, but this workshop will provide lab modules that include replication codes and teach students visualization and programming skills.
Prerequisites: n/a
Software: n/a
Fee: ICPSR Member - $1600; ICPSR Non-member - $2900
Immediate Regression Analysis: Linear Models and Extensions
Instructor: Scott Basinger, University of Houston (Department of Political Science)
Dates: May 30-June 2, 8:00 a.m. - 1:00 p.m. CST
Room: TBA; Hybrid
Course Description: This course extends the linear model to situations where independent variables' effects vary in interesting ways. Three main situations are covered: interactions between explanatory variables, nonlinearities, and limited dependent variables particularly counts and binary outcomes. After the course, students will be able to estimate, interpret, and draw curves that display the results of regression models with interaction terms, with quadratic and cubic functional forms, and with variables transformed by logarithms and exponents. Students will gain experience with Poisson regression, logit, and probit, and students will better understand these models' advantages and disadvantages. Students should start with a basic understanding of the least squares framework and assumptions. Previous experience with the R statistical computing software is helpful but the course includes labs providing all the code and teaching all the programming skills that students will need.
Prerequisites: n/a
Software: n/a
Fee: ICPSR Member - $1600; ICPSR Non-member - $2900