Gustavo Aguilar Works on Named Entity Recognition for Social Media
Gustavo Aguilar, a University of Houston Ph.D. student in computer science, was selected as a 2019 Snap Research Fellow. This fellowship, administered by Snap Inc., creator of the social media platform Snapchat, comes with a $10,000 award and a full-time paid internship. The fellowship program recognizes outstanding students carrying out research in areas relevant to Snap Inc.’s mission.
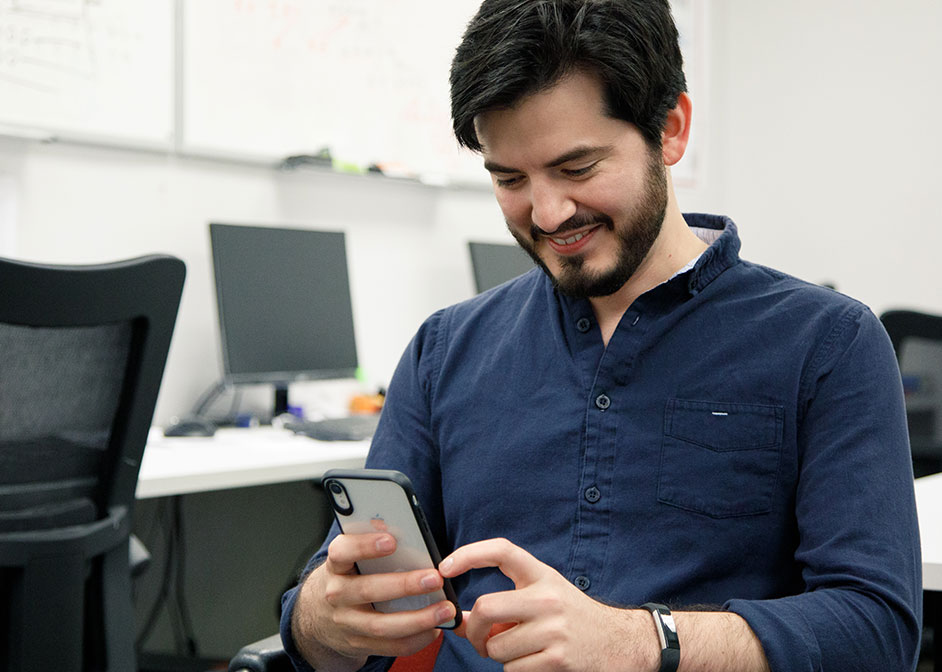
Aguilar, who entered graduate school in fall 2016, works in the field of natural language processing. His research is advised by Thamar Solorio, associate professor of computer science in the College of Natural Sciences and Mathematics. (Aguilar’s Website)
Natural Language Processing: Interaction Between Computers and Human Language
Natural language processing addresses the interaction between computers and human languages using machine learning and artificial intelligence. Some of the many examples of natural language processing include predicting sentiments in a text, converting written text into data, transcribing spoken words into text, translating passages from one language to another, formatting information into paragraphs, and correcting spelling and grammatical errors.
Virtual assistants, trained to recognize questions and generate answers, are a well-known application of natural language processing, found on smartphones or within the home.
Named Entity Recognition: Core Task Needed for Higher-Level Applications
Aguilar works on named entity recognition, which is one of the core tasks needed for many higher-level applications in natural language processing. Named entity recognition is used to recognize the people, places, organizations, and time within text. This way, phrases and sentences can be broken down into discrete entities, which help to better understand the meaning of text.
“The problem has been considered solved when it comes to news articles and well-written texts,” Aguilar said. “But when it comes to social media, performance metrics dramatically drop.”
Given all of the acronyms, abbreviations, and atypical uses of spelling and grammar, analyzing language in social media is exponentially harder. Adding to this complication is a lack of context, as social media posts are often brief, tailored to friends who can fill in the missing information.
“The context in social media is quite short, as opposed to the news, where you have a full article to explain a specific event,” Aguilar said.
Aspects of Aguilar’s work include working on entity disambiguation, as well as entity-level sentiment. Entity disambiguation is the correct recognition of entities within a statement. An example would be differentiating Houston the city, from Sam Houston the historical figure. Entity-level sentiment is about predicting the sentiments behind a statement, whether it be happy, sad, excited, or anything in-between.
Internship to Merge Thesis Research
Aguilar plans to intern with Snap Inc. in fall 2019, where his internship work is expected to overlap with his dissertation research.
“I like that this field is moving so fast, because it means we will eventually have a lot of breakthroughs,” Aguilar said. “Being part of these breakthroughs drives me most of the time.”
Aguilar was also the recipient of the computer science department’s 2018 Best Junior Ph.D. Student Award.
- Rachel Fairbank, College of Natural Sciences and Mathematics